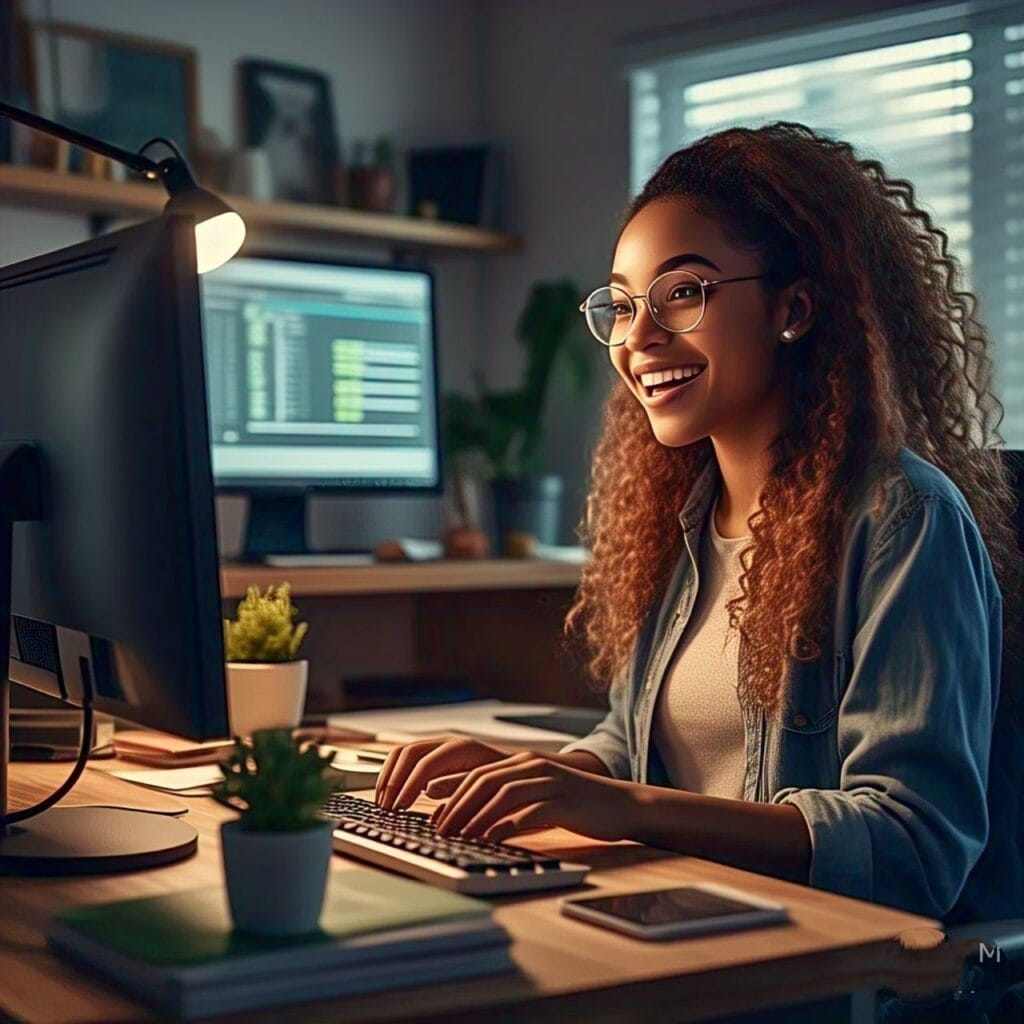
Agentic AI
Imagine AI that doesn’t just follow commands but actively solves problems, collaborates, and optimizes tasks without constant input. That’s Agentic AI—a major step forward from traditional AI. Unlike its predecessors, it operates with autonomy, capable of managing complex processes and making real-time decisions across industries. From automating workflows to transforming healthcare and finance, its potential is enormous. As organizations embrace these intelligent agents, the future of AI is becoming about more than just tools—it’s about partnerships.
What is Agentic AI?
Agentic AI represents the next phase in artificial intelligence, where systems operate with a level of independence that rivals human decision-making. It builds on the capabilities of generative AI but introduces advanced autonomy, enabling machines to not only learn but act and adapt dynamically. This category of AI goes beyond narrow tasks, involving strategic problem-solving, real-time adaptability, and self-governance.
Key Characteristics of Agentic AI
Agentic AI is defined by several key traits that make it a transformative step forward. Here are its most important characteristics:
- Autonomy: Unlike traditional AI, Agentic AI operates with minimal human supervision. It can analyze data, identify opportunities, and even take actions without constant user intervention.
- Logical Reasoning: These systems excel in applying advanced reasoning to complex scenarios. They don’t just process inputs—they consider context, weigh outcomes, and make informed choices.
- Enhanced Task Management: Agentic AI is capable of organizing and prioritizing workloads across multiple objectives, optimizing efficiency and ensuring goals are met seamlessly.
- Real-Time Adaptability: Perhaps its most impressive feature, Agentic AI can adapt to changing conditions instantly. Whether managing supply chain logistics during unexpected disruptions or assisting in disaster response, this flexibility sets it apart.
Agentic AI combines these capabilities to perform tasks that were once thought too complex for machines.
Agentic AI vs. Traditional AI Systems
How does Agentic AI compare to traditional AI? The differences are stark:
- Task Scope: Traditional AI systems are trained for narrow tasks—think chatbots or recommendation engines. Agentic AI, on the other hand, operates on goals and strategies rather than single-use cases. Platforms like UiPath highlight this distinction, focusing on adaptability and autonomous problem-solving.
- Data Dependency: Traditional AI systems often require extensive datasets to function efficiently. While these remain important for training, Agentic AI thrives on dynamic information and real-world inputs, processing in real time.
- Limitations: Traditional systems excel in controlled environments but struggle with unpredictability. Agentic AI is designed to handle uncertainty and shift focus when priorities change. For a deeper comparison of these AI paradigms, explore IBM’s comprehensive insights.
Agentic AI is a leap forward, offering flexibility and independence that traditional systems lack.
The Role of Small Language Models (SLMs)
In the evolution of AI, Small Language Models (SLMs) are playing a pivotal role. These compact alternatives to Large Language Models (LLMs) are uniquely suited to the kind of autonomy and adaptability that Agentic AI requires.
- Efficiency and Speed: SLMs are optimized for real-time applications. Unlike LLMs, they don’t require massive computational resources, making them faster and more accessible.
- Scalability: Their size allows for deployment in multi-agent systems, enabling several AI agents to operate collaboratively without overwhelming processing power.
- Relevance in Agentic AI: SLMs often serve as the communication foundations within Agentic AI systems, facilitating smooth data sharing and decision-making. For a breakdown of their impact, check out Forbes’ analysis.
As the focus shifts from massive datasets to nimble, efficient models, SLMs are proving to be indispensable tools in making Agentic AI a reality. Tools like AISera’s compact solutions highlight how SLMs are democratizing AI for broader use cases.
Agentic AI thus isn’t just about autonomy—it’s also about efficiency, scalability, and using the right tools to enhance performance.
Applications of Agentic AI in Industry
Agentic AI is reshaping industries with its ability to function independently, adapting to complex environments and executing tasks with precision. From healthcare to IT and beyond, its widespread adoption is unlocking new efficiencies and benefits. Below, we explore how this transformative technology is catalyzing progress across key sectors.
Healthcare Innovations
In healthcare, Agentic AI is addressing some of the most pressing challenges by enhancing patient care and improving workflows. This technology is capable of:
- Streamlining patient care through intelligent scheduling systems and automated administrative processes, freeing up time for medical staff.
- Improving medication administration by reducing errors via real-time monitoring and precise dose tracking.
- Facilitating personalized treatments using advanced data analytics to identify the best interventions for individual patients.
- Enhancing patient monitoring through wearable technology and AI-powered devices that interpret health data continuously.
Notably, AI systems are providing real-time support to healthcare professionals, enabling them to deliver high-quality care even in high-pressure situations. For an in-depth look at these applications, check out GE Healthcare’s insights.
Revolutionizing Financial Services
Agentic AI is taking financial services to the next level by improving decision-making, increasing efficiency, and enhancing security. Key applications include:
- Automation of repetitive processes like loan approvals, investments, and transaction monitoring.
- Cybersecurity enhancement, identifying threats in real-time and mitigating risks proactively.
- Portfolio management using dynamic systems that adapt investment strategies based on market changes.
- Personalized user experiences through tailored financial advice and customer service interactions.
These advancements are not only refining existing services but also enabling new opportunities through greater autonomy. Learn more about how Agentic AI is shaping banking and finance in this article by FinTechNews.
Impact on Networking and IT
Agentic AI is driving efficiency and innovation in networking and IT, making it a critical tool for organizations looking to stay competitive. Examples include:
- Operation optimization through predictive analysis and autonomous decision-making.
- Automating migrations to cloud services or updated architectures, reducing downtime and errors.
- Enhancing service levels by continuously monitoring infrastructures and suggesting improvements.
With the growing complexity of network systems, these benefits have become invaluable for IT leaders. For additional insights, see Cisco’s breakdown of Agentic AI’s potential.
The Emergence of Digital Twins
Digital twins—virtual replicas of physical systems—are becoming an essential training ground for Agentic AI. These virtual models enable industries to:
- Simulate real-world scenarios in a controlled environment for testing and predictions.
- Train AI systems with industry-specific data to improve accuracy and efficiency.
- Optimize operations by identifying inefficiencies before they manifest in physical systems.
For instance, manufacturing companies are using digital twins to replicate factory processes, allowing Agentic AI to identify bottlenecks and recommend solutions. To explore this further, read about how digital twins integrate with Agentic AI at Akira.AI.
By advancing these sectors, Agentic AI is shaping a future where industries operate seamlessly, efficiently managing complexities that would otherwise require significant time and effort.
Frameworks and Tools Enabling Agentic AI
As technology advances, creating a cohesive and efficient ecosystem for Agentic AI is essential. Building intelligent systems that act autonomously requires unified frameworks and specialized tools. These tools enable AI to process information, adapt in real time, and perform complex tasks across industries. Let’s explore the frameworks shaping this landscape.
Unified AI Stacks
Unified AI stacks are transforming how we deploy machine learning and generative AI while integrating agentic automation. These stacks provide a seamless way to combine multiple AI systems into a single, efficient framework.
- Why Unified Stacks Matter: Without a unified system, AI solutions often operate in silos, leading to inefficiencies. Using a centralized stack ensures that machine learning, data pipelines, and generative AI work together smoothly.
- Capabilities: Unified AI stacks facilitate better orchestration, enabling AI agents to learn, adapt, and manage workflows autonomously.
For an example of how unified AI stacks streamline operations, check out TechInformed’s take on integrated platforms for 2025.
Retrieval-Augmented Generation (RAG) Systems
RAG systems combine the power of large language models with real-time data retrieval, making them invaluable for Agentic AI.
- Enhancing Real-Time Decision-Making: These systems search external databases or datasets during conversations to provide up-to-date information, ensuring output relevance.
- Optimized Searches: Agentic AI benefits from RAG by avoiding static, outdated responses. Instead, it retrieves the latest data for accurate insights.
To dive deeper into RAG systems and their role in empowering Agentic AI, see Moveworks’ in-depth guide.
Specialized Agent Training
Not all AI systems need to handle everything. Training AI agents on niche, domain-specific datasets is becoming the norm for delivering tailored results.
- Why Domain-Specific Training Works: Generalized AI can miss critical details in specialized fields. Training on specific data ensures higher precision and greater relevance.
- Industries Benefiting from This Trend: Healthcare, legal, and financial sectors are already using domain-trained agents to handle industry-specific challenges effortlessly.
For more on tailored agent training, you can explore this detailed analysis from Scribble Data.
Each of these tools—unified stacks, RAG systems, and specialized training—represents a vital piece of the puzzle in advancing Agentic AI.
Challenges and Ethical Considerations
Integrating agentic AI into industries and everyday life brings a broad spectrum of promises—and challenges. As these systems become more autonomous, ethical concerns, security risks, and workforce readiness rise to the forefront. Here’s a closer look at the most significant challenges and how they shape the development and use of agentic AI.
Security and Trust in AI Systems
Agentic AI systems, much like any emerging technology, are vulnerable to security threats. These threats range from data breaches to manipulation of decision-making processes. The ability of these systems to operate autonomously amplifies concerns about safeguarding control.
- Data breaches and exploitation: Autonomous systems often handle sensitive data, making them prime targets for cyberattacks. A lack of robust defenses can lead to crises, from compromised medical devices to hacked autonomous vehicles. Learn more about the security risks in autonomous systems from this study.
- Trust deficits: Once compromised, restoring public trust in an AI system becomes a steep climb. For instance, cybersecurity failures in autonomous vehicles have even altered human trust dynamics, as observed in this research.
To address these risks, organizations must design security measures around the unique functionality of agentic AI, ensuring transparency and reliability.
Potential for Job Displacement
The rise in AI-driven automation continues to spark debates about its impact on employment. Agentic AI’s ability to independently perform tasks previously managed by humans presents both opportunities and challenges for the workforce.
- Critics argue that large-scale automation will displace jobs, especially in manufacturing, logistics, and support roles. As outlined by Smythos, employers must strike a balance between harnessing AI’s cost-saving potential and safeguarding jobs.
- Proponents highlight the chance for human-AI collaboration, suggesting that AI might eliminate repetitive tasks but also empower workers to focus on creativity and critical thinking.
The disruption caused by agentic AI demands a recalibration of how humans and machines collaborate in the workplace, revealing an evolving, but intricate, power dynamic.
Explainable AI for Risk Management
As agentic AI decisions grow more complex, ensuring these systems are explainable becomes critical. Explainable AI (XAI) promotes transparency, helping humans understand not only what decisions were made but also why they were made.
- Without explainability, oversight becomes nearly impossible, increasing the chances of negligence or unintended harm. For example, in risk-heavy industries like fintech, explainability can reduce errors and bolster compliance, as demonstrated by McKinsey’s insights.
- Transparency also fosters trust among stakeholders, creating a bridge between technical teams, regulators, and end-users. This understanding is essential to reduce fears over “black-box AI.”
By adopting XAI, organizations ensure accountability and minimize risks, keeping AI systems aligned with societal norms and expectations.
Skills Gap and Workforce Upskilling
For agentic AI to succeed, businesses must not only integrate these systems but also prepare their workforce for this shift. The growing AI skills gap presents a significant hurdle.
- A lack of technical expertise can hinder the adoption and effectiveness of agentic AI. Closing this gap means equipping IT and non-IT employees with the tools they need to work alongside AI. Recent reports, like those from HR Dive, reveal increased investment in workforce training to bridge this gap.
- Workforce upskilling programs focus on cultivating domain-specific knowledge, enabling employees to develop and manage AI responsibly. As highlighted by ITPro’s analysis, this shift involves retraining on the use of AI tools and fostering a better understanding of ethical AI implementation.
Without proactive efforts to upskill the workforce, organizations risk creating a divide between those who benefit from agentic AI and those left behind.
These challenges underline the need for a balanced approach as agentic AI is scaled across industries. While the road ahead presents obstacles, addressing them proactively will be the key to unlocking its full potential.
Future of Agentic AI
As we look ahead, the potential of Agentic AI is not just about improving existing technology; it’s about creating entirely new paradigms for interaction and efficiency. Understanding its future implications involves exploring three critical areas: the rise of superagents, increased commercial adoption, and the evolving landscape of AI governance.
The Rise of Superagents
The next frontier in Agentic AI is the emergence of superagents—intelligent systems that can orchestrate various functions and processes across multiple domains. These superagents are capable of combining data from diverse sources and executing complex tasks that traditionally required human oversight. Imagine AI agents that can manage supply chains, interact with customers, and analyze market trends, all while learning and adapting in real time.
- Integrative Intelligence: Superagents analyze data from multiple inputs, including customer interactions and inventory levels, making decisions that enhance operational efficiency. This capability positions them as invaluable assets in industries like finance and healthcare, where timing and precision are crucial. Learn more about superagents in this Forbes article.
- Real-World Problem Solving: Designed to tackle messy, multilayered issues, superagents are expected to revolutionize how organizations approach problem-solving. With their ability to interpret complex data and environments, they promise to boost productivity and innovation at an unprecedented scale. For insights into this breakthrough, check out this Axios article.
Increased Commercial Adoption
The commercial sector is rapidly embracing Agentic AI, with projections indicating substantial growth in its deployment. Organizations are beginning to recognize the competitive advantage that these autonomous systems can provide.
- Forecasts for Adoption Rates: By the end of 2025, a significant percentage of enterprises utilizing generative AI will transition to Agentic systems, reflecting a serious commitment to modernizing operations. Reports suggest that up to 25% of enterprises could be deploying these systems, according to a recent forecast by BCG.
- Sector-Specific Benefits: Industries like finance are already experiencing the transformation Agentic AI brings through automation and enhanced customer service capabilities. This technology is changing the game for how institutions manage tasks from loan processing to risk assessment. Discover additional details on its impact in FinTech Futures.
Evolving AI Governance
As Agentic AI becomes more ingrained in business and society, the need for robust governance frameworks grows. Ensuring that these technologies are deployed ethically and responsibly is paramount.
- Framework Necessity: With the rapid expansion of AI capabilities, frameworks guiding its ethical use are essential. Organizations and governments are developing guidelines to oversee AI deployments, aiming to mitigate risks associated with decision-making autonomy. For a deep dive into governance trends, explore the World Economic Forum’s findings on AI governance.
- Focus on Collaboration: Future governance will not rely solely on strict regulations but will involve fostering collaboration among technology developers, businesses, and policymakers. This approach aims to balance innovation with ethical responsibilities. Insightful discussions on this topic are available in IBM’s thoughts on governance evolution.
The future of Agentic AI stands as a pivotal moment, promising innovations through superagents, widespread adoption in commercial practices, and an evolving governance landscape. These elements will undoubtedly shape how we interact with technology in the years to come.
Conclusion
Agentic AI holds immense potential to reshape industries, enhancing autonomy and efficiency across the board. Its ability to operate independently and make real-time decisions sets it apart from traditional AI models. However, the journey isn’t without hurdles. Ethical issues, security concerns, and workforce implications must be carefully navigated to ensure responsible use.
As we embrace the capabilities of Agentic AI, organizations need to foster collaboration between humans and machines. This partnership could unlock new levels of productivity and innovation.
What will you do to prepare for this changing landscape? Share your thoughts below, and let’s keep the conversation going!